How to Mitigate Generative AI Security Risks & Compliance Issues in 2025
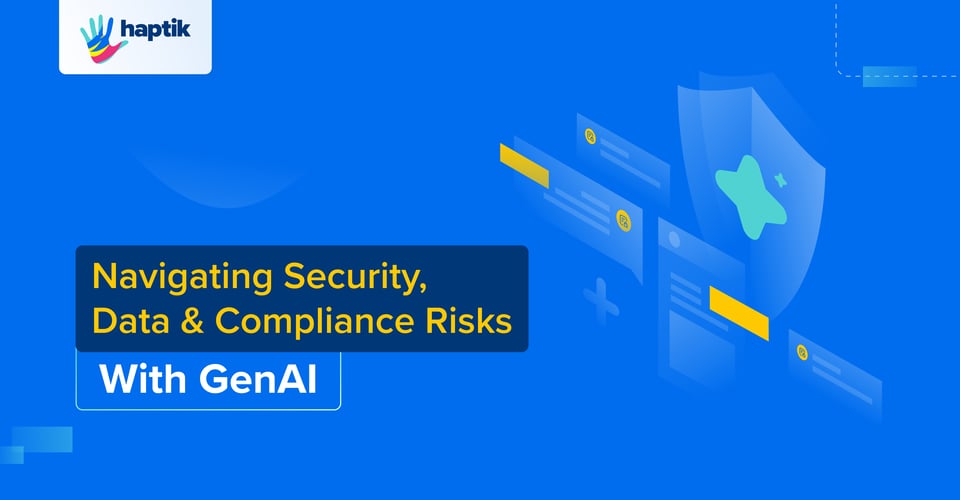
Generative AI is transforming businesses across industries, unlocking efficiency and innovation at scale. Its disruptive capabilities help streamline processes such as content generation, image generation, and data analysis, among others. However, along with its tremendous potential, GenAI brings many inherent risks in security, data protection, and compliance for companies Navigating this risk landscape presents a huge dilemma: how can they leverage GenAI’s disruptive power while protecting sensitive information and adhering to the evolving data protection laws?
In this blog, we explore the key risks associated with GenAI adoption and provide insights into best practices for mitigating GenAI risks. We will focus on critical issues beyond immediate concerns like cost, choice of GenAI provider, or the vast array of Large Language Models (LLMs) available. Our focus will center on pressing challenges such as data leakage, model bias, regulatory compliance, and the essential steps to ensure GenAI deployments remain secure, compliant, and ethical. By following these practices, businesses can adopt GenAI responsibly, unlocking its potential while minimizing risks.
1. Security Risks: Protecting Sensitive Data in AI Models
One of the primary concerns with GenAI lies in the security of data used to train these models. Since GenAI models often require vast amounts of data for training, they may inadvertently expose sensitive information or, if poorly managed, provide an entry point for cyber threats.
Key security risks:
- Data leakage: GenAI models can reveal sensitive information from their training datasets. For instance, if a model was trained on confidential data, it might generate outputs that expose private information. This risk is particularly relevant in sectors like healthcare and finance, where data sensitivity is paramount.
- Model vulnerabilities: GenAI models can be subject to adversarial attacks, where malicious inputs deceive the model into producing unintended or harmful outputs. Such vulnerabilities can be exploited by bad actors to gain unauthorized access to systems or data, potentially compromising organizational security.
- Increased attack surface: Integrating GenAI into business operations introduces new endpoints that may lack robust security controls, expanding the organization’s attack surface and potentially exposing it to cyber threats.
Mitigation strategies:
- Data anonymization: Organizations should anonymize data before using it for training AI models, ensuring that sensitive information is not directly exposed within the model.
- Secure model training: By implementing strict access controls and encryption during model training, companies can reduce the risk of data leakage.
- Regular security audits: Regular audits of AI models and their usage can help identify potential vulnerabilities and prevent adversarial attacks.
2. Data Protection Risks: Addressing Privacy Concerns
Data protection is a significant concern with GenAI, especially as data privacy regulations become stricter worldwide. GenAI models often use personal data in their training, raising potential concerns about user consent and privacy compliance.
Privacy concerns:
- Data minimization and purpose limitation: Under regulations like the GDPR, organizations are required to collect only the minimum data necessary and use it for specific, lawful purposes. However, the sheer volume of data required for training GenAI models can conflict with these principles.
- Inference of personal information: GenAI models trained on large datasets might infer and even generate personal details without explicit user consent. This could lead to unauthorized exposure of personal data, breaching data protection regulations.
Compliance considerations:
- User consent: Organizations must obtain clear, explicit consent from users whose data might be used in AI training, and they should clearly inform users about how their data will be utilized.
- Right to be forgotten: If a user requests data deletion, it may be challenging to ensure that their data is completely removed from the training dataset without extensive model retraining, creating potential compliance gaps.
- Data provenance: Establishing clear data lineage and provenance is crucial to understanding the sources of data used in training, ensuring that data used is compliant with regulatory requirements.
Mitigation strategies:
- Data governance framework: A robust data governance framework helps manage data throughout its lifecycle, ensuring compliance with data protection laws.
- Synthetic data: Using synthetic data instead of real personal data can help train GenAI models while minimizing privacy risks.
3. Compliance Risks: Navigating Complex Regulatory Landscapes
As regulatory bodies continue to scrutinize AI, compliance is becoming a significant concern for companies adopting GenAI. Regulations are emerging to ensure ethical AI use, transparency, and accountability. The EU's AI Act and proposed U.S. legislation on AI are just two examples of the increasingly complex compliance landscape that GenAI must navigate.
Compliance challenges:
- Transparency and explainability: Regulations require that AI decisions be explainable and transparent. However, many GenAI models, especially deep learning-based models, are complex and function as "black boxes," making it challenging to trace how specific outputs are generated.
- Accountability and liability: Determining accountability when an AI-generated output leads to adverse outcomes can be complex. GenAI’s autonomous nature poses questions around liability, especially in high-stakes fields like healthcare or finance.
- Cross-border data transfers: Companies using GenAI models trained in different jurisdictions must be cautious about cross-border data transfers, as this could lead to regulatory non-compliance if data privacy requirements differ between regions.
Mitigation strategies:
- Model explainability: Organizations should strive to make their GenAI models as transparent as possible, using techniques like model interpretability tools to comply with regulatory requirements.'
- Compliance frameworks: Adopting AI compliance frameworks, such as those offered by ISO/IEC (e.g., ISO 42001 for AI Management System and ISO 27001 & 27701 for information security & privacy management respectively), NIST AI 6001 and EU AI Act of 2023 can provide a structured approach to addressing regulatory compliance.
- Ongoing monitoring and reporting: Regular compliance monitoring and reporting help organizations stay aligned with evolving regulations and demonstrate due diligence to regulators.
4. Ethical and Bias Considerations in GenAI
Generative AI models are prone to reproducing biases present in their training data. This can lead to unethical or discriminatory outputs that may damage brand integrity and expose the organization to compliance risks:
Bias propagation
- Models trained on biased data may generate outputs that reinforce stereotypes or exhibit bias against certain groups, which can be both legally and reputationally damaging.
Ethical concerns
- Misuse of GenAI for deceptive practices, such as deepfakes or misinformation, raises ethical issues and may draw regulatory scrutiny, especially if such content harms consumers or breaches consumer protection laws.
Mitigation strategy
- Organizations should implement bias detection and mitigation tools, conduct fairness audits, and prioritize diverse data representation in training datasets. Ensuring GenAI outputs align with ethical guidelines can mitigate both legal and reputational risks.
5. The Need for Responsible GenAI Adoption
GenAI’s potential to drive innovation and efficiency is undoubted, but so are its security, data-protection, and compliance risks. To leverage its benefits responsibly, businesses must adopt a proactive approach, balancing innovation with ethical-considerations and regulatory compliance.
Related: [Download] The CXO’s Guide to Enterprise Gen AI Adoption
By embedding security, privacy, and compliance into the design and development of GenAI systems, businesses can navigate the complexities of this powerful technology. This ensures that they remain competitive and compliant, securing their position in an increasingly AI-driven landscape. The double-edged sword of GenAI demands both vigilance and vision, empowering businesses to leverage its capabilities responsibly and sustainably.
Also Read: Securing PII Data at Scale