This article has been co-authored by Shrey Jain (Sr. Product Manager, Haptik) and Gaurav Arora (Machine Learning Engineer, Haptik).
With the COVID-19 pandemic causing a surge in support volumes globally, Intelligent Virtual Assistants (IVAs) are fast becoming the go-to choice for the businesses to cater to support requests. Enterprises are evaluating multiple Natural Language Processing (NLP) based IVA vendors to achieve automation, but feel perplexed in making a choice amongst a plethora of vendors, with each vendor claiming to have the ‘highest automation’ or ‘highest cost savings’. However, there is more to the IVA story than automation, with a key aspect being how an IVA handles “Out-of-scope queries”.
Most enterprise-oriented IVAs are trained for a fixed set of use-cases, called the SCOPE of the IVA. The IVA is expected to answer user queries falling in-scope and not answer user queries that are out-of-scope.
Here’s a visualization of both the concepts:
However, customers that visit the website or mobile app may/ may not have prior knowledge about the IVA’s scope. It’s reasonable to expect users to ask certain queries which are outside the IVA’s SCOPE.
Thus, while evaluating a conversational AI vendor, businesses should pay adequate attention to the robustness of the NLP model and its ability to identify out-of-scope queries.
It is a good idea to always keep in mind these words:
“A smart IVA not only knows what it knows, but also knows what it doesn’t know.”
Why it’s important for an IVA to not answer Out-of-Scope Queries
Answering out-of-scope queries by misidentifying them as in-scope, can potentially harm customer experience. Since an IVA also serves as an official communication channel for the brand, it may cause customer churn or reduce brand credibility.
Listed below are a few instances showing the dent caused by IVAs that are unable to identify out-of-scope queries and continue to reply to the user.
For instance in the above example, the IVA was trained to answer queries on abortion laws of USA. However, it couldn’t recognise that the user’s query is regarding Indian laws and ended up giving a fatally wrong answer.
Furthermore, answering out-of-scope queries may also cause a negative impact on the NPS or may cause loss in business opportunities, as seen in the example below. If the IVA is not able to flag out-of-scope queries as such, an enterprise may never realize loss of potential customers or a detrimental impact on their experience.
At Haptik we understand the gravity of rightly comprehending IVA scope and the immense damage caused by an IVA that answers out-of-scope queries. Thus, while building and implementing our NLP algorithms, we put a distinct and intentional focus on correct identification of out-of-scope queries.
Recently at EMNLP 2019, one of the most prominent conferences on NLP, a publication evaluated a few platforms and models on their performance in identifying out-of-scope queries. Most vendors present in the industry use one or a combination of these models to power their IVAs.
The evaluation was based on SENSITIVITY i.e Out of 100 out-of-scope queries received by the IVA, how many were correctly identified by the IVA as out-of-scope.
GRAPH 1 shows the performance of various models and platforms when adequate quantity of Training Data has been used to test the performance of the IVA.
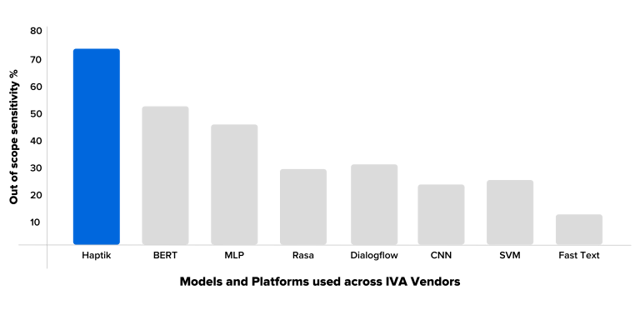
GRAPH 1- Sufficient Training Data
GRAPH 2 shows the performance of various models and platforms when Training Data is reduced to a sixth of the original Training Data.
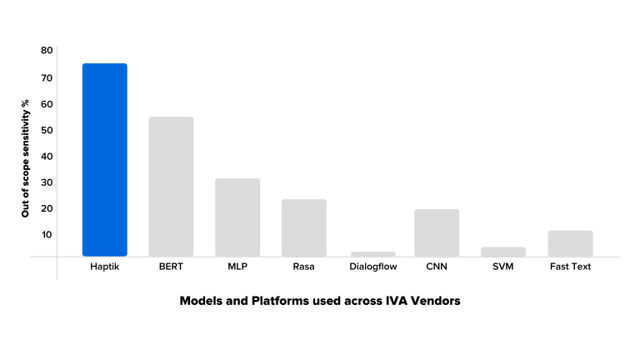
LEARN MORE: How Virtual Assistants Enhance CX with Clearer Conversations
How Haptik’s NLP model helps our IVAs deliver exceptional performance
-
Haptik’s product philosophy has been mastered through 7 years of building IVAs, not only for other enterprises but also for Haptik’s own B2C application over many years. To achieve the same, Haptik’s NLP model uses a combination of multiple models to process and answer a user’s query. This enables the IVA to simultaneously achieve a word level and a contextual understanding of the user’s query.
-
Haptik trains its IVAs on the negative user queries as well (in NLP parlance, adversarial queries).
a. E.g For a user utterance Please book a flight for me, a negative user utterance would be Please sell a flight for me or Please book a train for me. -
Haptik’s NLP Model trains the model on negative utterances by varying important keywords in positive utterances. This enhances the model’s accuracy to identify and truly understand user intent, and also become equally robust on what not to identify.
Thus, when businesses evaluate the NLP Models of the IVA vendors, they should pay distinct focus on the performance on out-of-scope queries.To conclude, let us return to these words:
“A smart IVA not only knows what it knows, but also knows what it doesn’t know.”
Want to develop an Intelligent Virtual Assistant solution for your brand?