Most businesses today are trying to implement a conversational solution to help improve overall Customer Experience. And if you are a Customer Experience or Digital Transformation professional looking for one, you might be a little confused about the difference between chatbots and intelligent virtual assistants, among a host of other terms used for such solutions.
Intelligent Virtual Assistant (IVA) is a term that has entered common usage over the past year as a means of discerning ‘good chatbots’ vs’ bad chatbots’. Broadly speaking, the term ‘chatbot’ is typically used for a solution that can handle only simple, routine queries and FAQs. ‘Intelligent Virtual Assistants’, on the other hand, are more advanced conversational solutions – equipped with NLU (Natural Language Understanding), NLG (Natural Language Generation), and Deep Learning, that enables them to understand and retain context and have more productive conversations with users.
While the terms ‘chatbot’ and ‘Intelligent Virtual Assistant are still sometimes used interchangeably by customers and businesses alike, there are significant differences between the two in terms of scope, complexity, and capability, all of which are far greater in IVAs as compared to chatbots. Chatbots can simulate a conversational experience to a certain extent but are ultimately constrained by having to work off a limited script – lacking the ability to learn over time and adapt to context. IVAs, on the other hand, have the advanced capabilities to truly serve ‘assistants’ to customers – emulating human interaction while carrying out a wide variety of tasks to fulfill a user’s requirements.
Learn more about the fundamental distinction between a chatbot and an Intelligent Virtual Assistant.
Buzzwords and hyperbole aside here’s a quick set of actual examples to tell apart a chatbot and an Intelligent Virtual Assistant based on their capabilities:
Negations
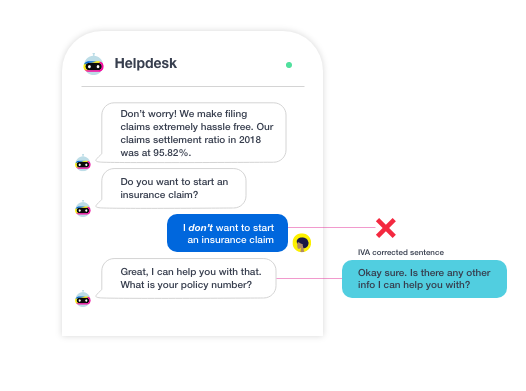
Start an insurance claim vs. Don’t start an insurance claim
In the above example, the meaning of the sentence has completely changed with the addition of a single word in a key place. Simple chatbots that use basic sentence similarity models can’t tell the difference between these two sentences. A chatbot, which has basic word matching, would not be able to detect the difference between the two sentences and will simply start the flow that is best matched to the sentence. An IVA however can detect negations or other nuances in language, and thus responds to the specific intent of the customer with greater precision. This capability reduces false positives by 5%.
Disambiguation
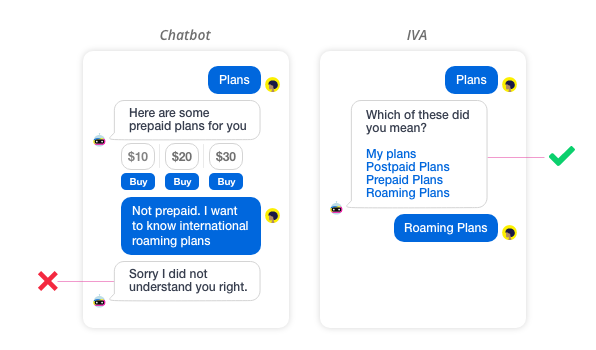
Sometimes the content of the query sent by the user is simply not enough for the IVA to understand their intent. What would an actual person do in such a situation? They’d ask a question to clarify. That’s precisely what any good IVA should do. It should clear out the ambiguity around the query from the user. For instance, while interacting with an IVA, consumers may just say “Plans” but since the intent behind this is not clear an IVA will respond to clarify the intent by suggesting close matches such as “Show existing plans”, “Browse new plans”, “Postpaid Plans” etc. Disambiguation has been found to significantly increase the overall automation levels of IVAs, from around 70% to as high as 96%.
READ MORE: How Chatbots Enhance CX with Clearer Conversations
Vocal Disambiguation
Didn’t hear you correctly vs. Didn’t understand what you said
As IVAs expand to the realm of voice, a lot of developers are trying to replicate the chat experience over voice-based platforms. This is a complex task for a number of reasons. While factors such as spelling mistakes are what affect the written word, on the voice you have different errors such as background noise or phonetic errors. Moreover, as we move past geographies, the accents of the consumers vary widely which adds to the complexity. An IVA’s algorithms are trained to recognize such specifics in speech, whereas a chatbot would simply translate from one language to another and send a generic response (that would likely include errors) based on the best match. This nuance in the engine helps reduce user drop-offs by 15% on average.
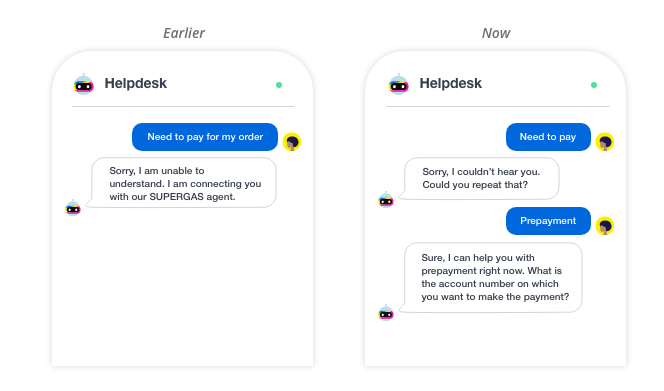
In the above example, the IVA is able to prompt the user by detecting that something is missing from the context or intent.
Spelling Mistakes & Slangs
There can be multiple variations behind spelling mistakes and those variations in turn could lead to multiple intents or interpretations of the message. While a chatbot would throw the generic response based on the best match, an IVA would be equipped with an effective spell correction model that would detect such mistakes.
A well-designed IVA leverages multiple spell correction models to detect different types of spelling mistakes/variations and resolve them accordingly.
Phoneme Model: Handles spelling mistakes that occur due to wrong usage or omission of vowels in a word. For e.g. avalablity is corrected to availability.
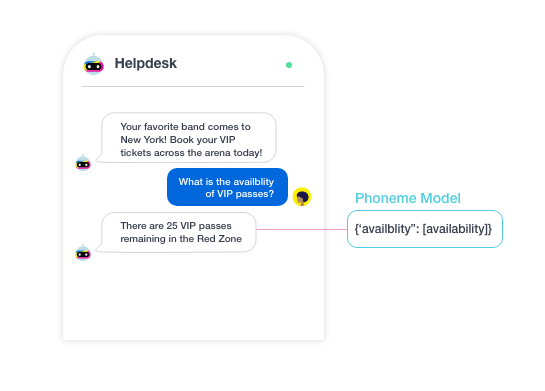
Symspell Model: Handles qwerty-based errors which unintentionally occur while typing from the keyboard. For e.g. adjacent character errors, wherein a character is accidentally replaced by a nearby character on the keyboard (training becoming trsining due to ‘a’ being replaced by ‘s’).
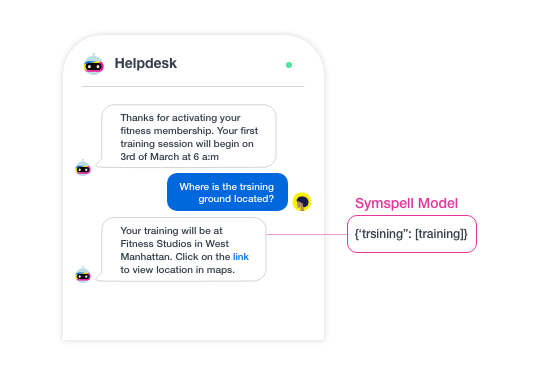
Context Model: Determines the most probable word from the list of suggested words for misspelled words from the Symspell and Phoneme models. For e.g. in the case of the sentence “I wnt to buk flight”, there are two mispelt words with multiple suggestions – wnt (‘went’, ‘want’) and buk (‘book’, ‘buy’). The model will calculate the probabilities of each of the sentence variations matching the user intent and selects the sentence with the highest probability.
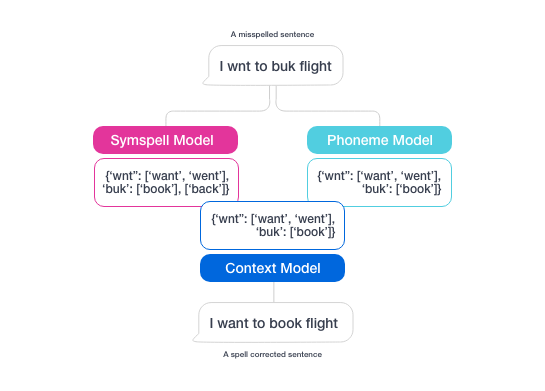
With domain-specific training (which helps the IVA in getting more details and general knowledge of the domain) and contextual corrections (which helps the IVA in understanding the context of the query within a domain), an IVA can pinpoint the right intent and take appropriate action without interrupting the customer experience. When it comes to voice, IVAs have built-in phoneme normalization, which helps in detecting the right text from a user’s speech by minimizing the errors due to speed of speech, and accents among other things.
Paraphrases for Intents
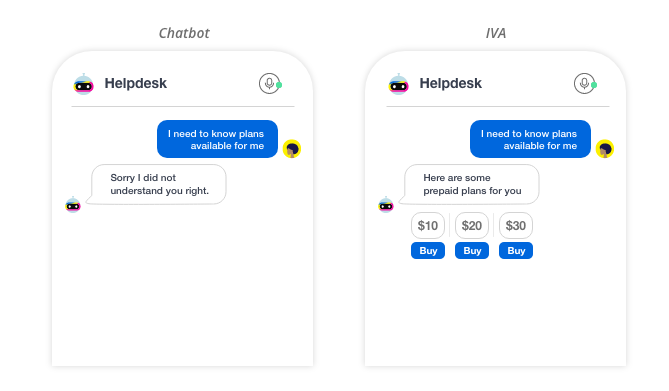
In our everyday language, we tend to express the same intents using a combination of multiple words. A good IVA should be able to detect the same intent using all possible combinations of words. For example, if the IVA is trained using the input “Show me internet plans?”, it should be able to answer “I need to know plans available for me”.
Intents with Minor Differences
‘Show my internet plan’ vs. ‘show me internet plans’
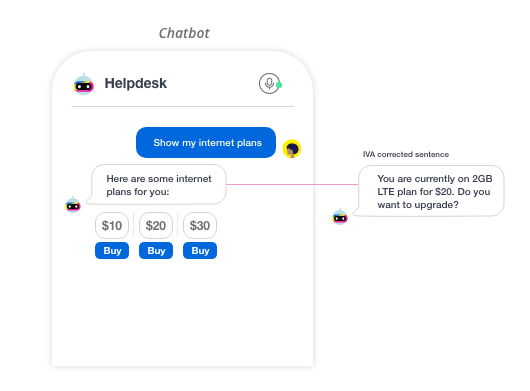
The intents look very similar on the surface, but there is in fact a significant difference. In the first case, the user wants to check what his current plan is, as opposed to the second case, where the user wants to see all available plans. A good IVA would be able to detect the nuances between the two.
Out-of-Scope Questions
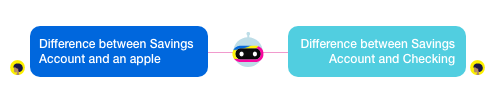
A chatbot would normally match “Difference between Savings Account and an apple” to “Difference between Savings Account and Checking”
This is because a simple sentence similarity check would consider them to be very close sentences, due to there being only a one-word difference. However, that one word difference completely changes the intent. An IVA should be able to handle these nuances. The ability to effectively address out-of-scope questions reduces false positives by 5%.
Understanding Long Sentences
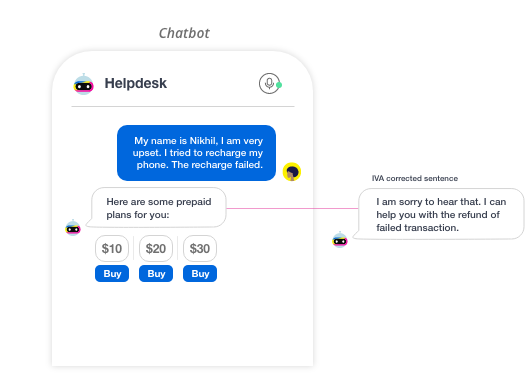
A lot of the time users tend to add extra historical information in the query which makes it difficult for a usual chatbot to understand. An IVA on the other hand has the ability to extract the relevant intent and entity from the message and ignore the information which is not required.
Retaining Context
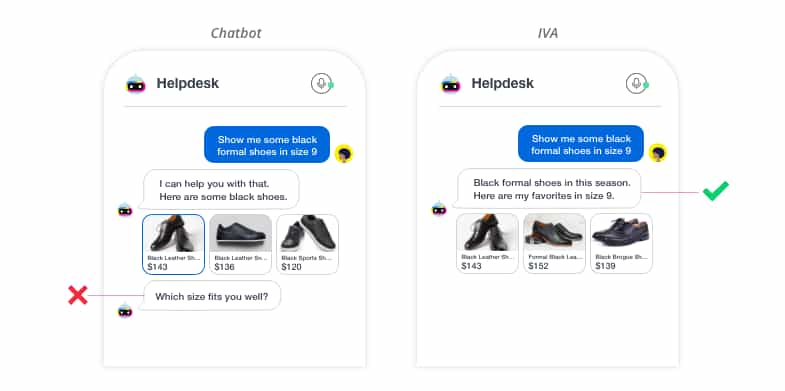
An IVA is able to retain context after a few messages are exchanged whereas a chatbot is not advanced to keep up with the information provided by the user in earlier messages. In the example below, the IVA is able to detect that the user is looking for a particular size.
Conclusion
A conversational solution that has the ability to understand and retain context, account for nuances in language, and effectively deal with ambiguity can be considered an Intelligent Virtual Assistant, rather than a simple chatbot. With these capabilities and more, IVAs have the ability to truly put a customer’s needs at the forefront – significantly enhancing a brand’s customer experience and ultimately driving ROI.
